Artificial intelligence (AI) has gained immense significance in the realm of metal manufacturing, transforming the industry by optimizing efficiency and productivity. This blog focuses on the use of AI in manufacturing, where AI technologies play a central role in optimizing operations and achieving higher levels of quality and performance. From collaborative robots working alongside human workers to intelligent predictive maintenance systems, these AI-driven solutions have the potential to drive growth, increase competitiveness, and meet the evolving demands of the industry.
In the ever-evolving landscape of technological advancements, it is essential to acknowledge the rapid pace at which artificial intelligence (AI) is advancing. As we recognize that the information presented in this blog may quickly become outdated. Nonetheless, armed with the knowledge of AI’s swift progress, we embark on a comprehensive exploration of its transformative applications in reshaping the metal manufacturing industry.
By delving into real-world examples, case studies, and industry-specific references, we aim to provide a comprehensive understanding of how AI is transforming component manufacturing. Embracing AI in this domain unlocks new opportunities for efficiency, quality, and innovation, and we invite you to join us on this journey as we uncover the common AI use cases in metal manufacturing and highlight the transformative impact of AI technology on the industry.
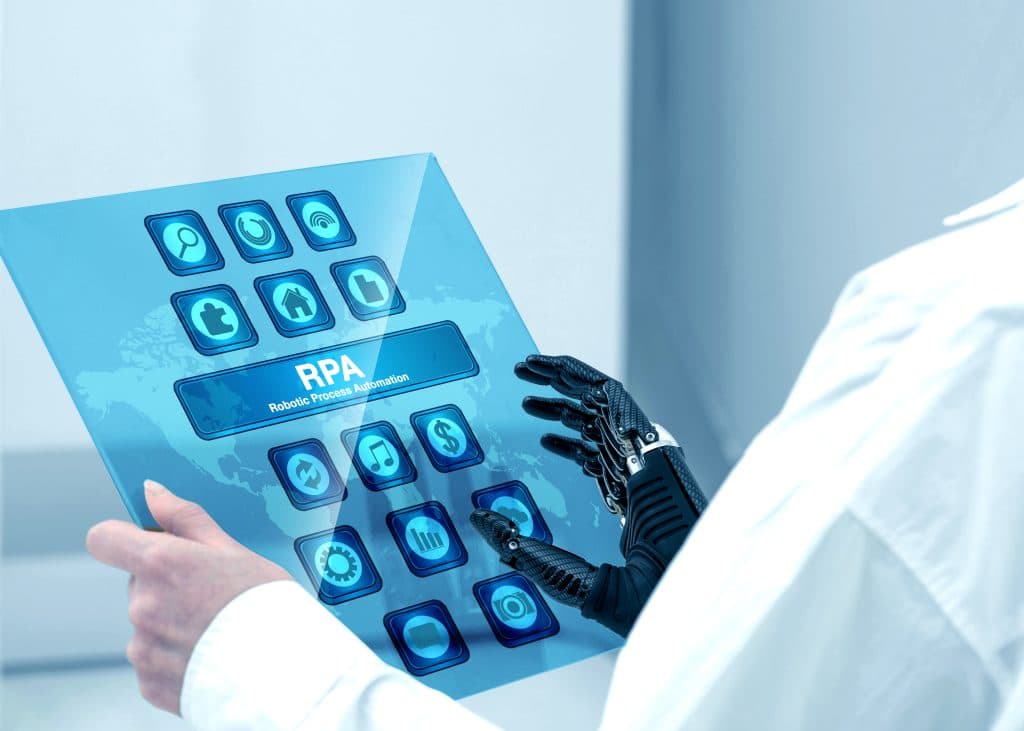
Digitalization In Manufacturing
The manufacturing landscape is experiencing a profound upliftment due to the pervasive influence of digitization. Companies across various industries are actively seeking to revolutionize their ecosystems by embracing innovative technologies.
The adoption of game-changing solutions within the realm of Industry 4.0 has emerged as a defining characteristic of digital leaders. These organizations are at the forefront of leveraging cutting-edge advancements to drive unprecedented transformation and success.
- IoT in Manufacturing Industry
The manufacturing industry is undergoing a profound transformation with the advent of the Internet of Things (IoT), marking the arrival of an era characterized by interconnected devices and intelligent systems. IoT is driving significant changes by seamlessly integrating physical objects with sensors, software, and network connectivity. This integration enables devices and systems to communicate, collaborate, and exchange data, leading to unprecedented opportunities for innovation and optimization in manufacturing processes.
With IoT, manufacturers can harness the power of real-time data, enabling them to make informed decisions, enhance operational efficiency, and deliver higher-quality products. The ability to connect and control various devices and systems brings about a new level of intelligence, enabling manufacturers to optimize production, improve supply chain management, and drive overall productivity. The transformative potential of IoT in the manufacturing industry is reshaping traditional approaches and unlocking new possibilities for growth and competitiveness.
- Industry 4.0 optimises computerization of industry 3.0
In the era of Industry 4.0, the landscape of manufacturing undergoes a remarkable transformation, driven by the interconnectedness and communication capabilities of computers. Unlike the disruptive introduction of computers in Industry 3.0, where they added a new technology layer, Industry 4.0 takes it a step further by enabling computers to autonomously make decisions without human intervention.
This advancement is made possible by the convergence of cyber-physical systems, the Internet of Things (IoT), and the Internet of Systems, which collectively pave the way for the realization of smart factories. As machines become smarter through the acquisition of vast amounts of data, our factories are poised to become more efficient, productive, and sustainable. The true power of Industry 4.0 lies in the networked collaboration and information sharing among digitally connected machines.

Common Use of AI in Manufacturing
1. Cobots work with Humans
Cobots, short for collaborative robots, are a specialized type of robotic technology that work in close proximity to human workers, complementing their capabilities and functioning as an extra set of hands. What sets cobots apart is their versatility and adaptability in learning various tasks, making them valuable assets in the metal manufacturing industry. Unlike traditional autonomous robots, cobots possess spatial awareness, enabling them to detect and avoid obstacles in their environment, allowing them to seamlessly collaborate with human workers.
In metal manufacturing, cobots find wide-ranging applications due to their ability to handle heavy lifting and perform tasks on assembly lines. For instance, in automotive factories, cobots are instrumental in lifting heavy car parts and holding them in place while human workers secure them, thereby reducing the risk of injuries and enhancing overall efficiency. Additionally, cobots excel in tasks that require precise assembly, ensuring accuracy and consistency throughout the production process. These cobots are capable of learning and adapting to different components and assembly techniques, contributing to increased productivity and quality in metal manufacturing.
The collaborative nature of cobots, combined with their ability to learn and adapt, has made them indispensable in various manufacturing sectors. They enhance productivity by enabling human workers to focus on more complex tasks that require critical thinking and decision-making, while the cobots handle repetitive and physically demanding operations. This partnership between human workers and cobots not only improves overall efficiency but also creates safer working environments and minimizing physical strain.
2. Robotic Process Automation (RPA) tackles tedious tasks
In the realm of metal manufacturing, Robotic Process Automation (RPA) software has emerged as a powerful tool, revolutionizing operations and driving efficiency. With its ability to automate repetitive tasks and handle data processing, RPA software proves particularly advantageous in streamlining back-office operations within the manufacturing sector.
RPA software serves as a catalyst for efficiency by automating tasks that were previously time-consuming and prone to errors. For instance, in order processing, RPA software eliminates the need for manual data entry, ensuring accuracy and reducing processing time. This automation not only saves valuable resources but also minimizes the risk of delays in the supply chain. Manufacturers can leverage RPA software to generate purchase orders, track inventory levels, and manage shipments, facilitating seamless supply chain management.
Moreover, RPA software acts as a bridge between different systems and applications, enabling smooth data integration and communication across departments. In quality control processes, RPA software extracts data from various sources, performs calculations, and generates real-time reports, empowering manufacturers to make prompt decisions based on accurate. By automating these data-intensive tasks, RPA software enhances operational efficiency and optimizes overall performance.
3. Digital twins help boost performance
Digital twins have emerged as a transformative technology, developing the way companies optimize their machinery and improve performance. Digital twins are virtual replicas of physical objects that leverage smart sensors and AI technology to provide real-time insights into the condition and efficiency of machinery throughout its lifecycle.
Employing the power of digital twins, manufacturers gain a comprehensive understanding of their equipment’s performance and maintenance needs. These virtual replicas enable real-time monitoring of key performance metrics such as temperature, vibration, and energy consumption. Through advanced AI analytics, manufacturers can detect patterns, identify anomalies, and predict maintenance requirements, facilitating proactive interventions to prevent breakdowns and optimize production processes.
Implementation of digital twins extends across various industries, including metal manufacturing. For example, in the aviation industry, digital twins of airplane engines receive data from smart sensors during take-off and landing, enabling airlines and manufacturers to closely monitor performance, anticipate potential issues, and schedule timely maintenance. This proactive approach not only enhances safety but also maximizes operational efficiency, minimizing costly downtime.
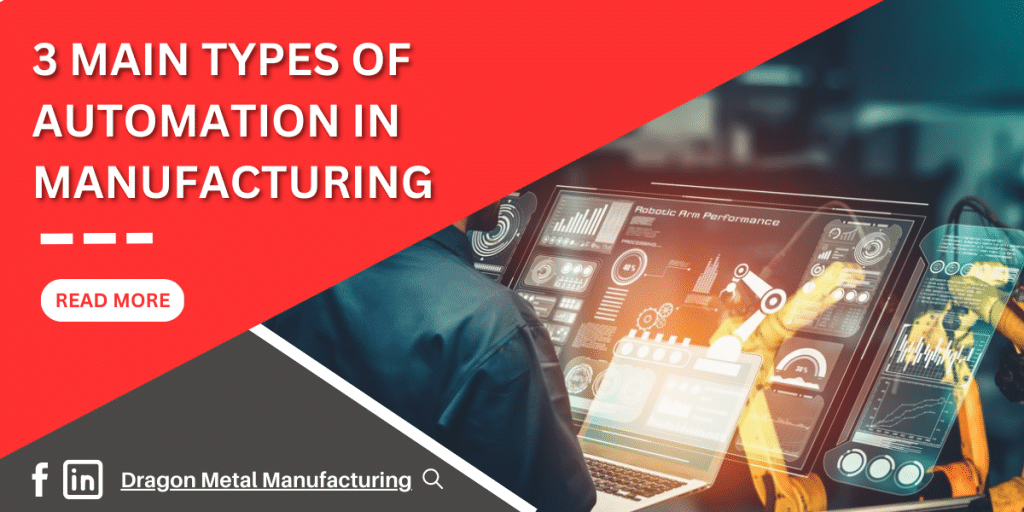
4. Predictive maintenance improves safety and lowers costs
Proactive maintenance is vital for ensuring worker safety and optimizing operational costs. One approach that has gained prominence is AI-based predictive maintenance, which leverages machine learning algorithms to anticipate servicing needs and minimize unplanned downtime.
Predictive maintenance systems analyse real-time data from sensors and equipment to detect patterns, anomalies, and early signs of potential failures. By monitoring the condition of machinery and predicting maintenance requirements, manufacturers can take proactive measures to address issues before they escalate, reducing the risk of accidents and optimizing the lifespan of equipment.
Implementing predictive maintenance brings several benefits to manufacturing processes:
- Enhanced worker safety: By identifying potential equipment failures in advance, predictive maintenance systems enable manufacturers to schedule maintenance activities during planned downtime, reducing the likelihood of sudden breakdowns that could endanger workers or cause accidents.
- Cost reduction: Predictive maintenance helps optimize the allocation of resources by identifying the precise timing for maintenance interventions. By avoiding premature or unnecessary maintenance, manufacturers can save on labour costs, reduce inventory expenses, and prevent production losses caused by unplanned downtime.
- Improved equipment performance: By proactively addressing maintenance needs, predictive maintenance systems help maintain machinery in optimal condition, ensuring consistent performance and minimizing disruptions in production.
- Efficient spare parts management: By predicting servicing needs, manufacturers can accurately plan their spare parts inventory, ensuring the availability of critical components when required, and minimizing delays in maintenance activities.
For instance, in the manufacturing of heavy machinery, predictive maintenance systems can analyse data from sensors embedded in equipment and detect anomalies indicative of potential failures. By identifying the need for maintenance in advance, manufacturers can schedule repairs during planned maintenance windows, reducing the risk of costly breakdowns and optimizing the safety of workers.
5. Machine learning algorithms predict demand
Machine learning algorithms have become invaluable in the manufacturing industry for their ability to analyse consumer behaviour, identify buying patterns, and forecast demand. By leveraging vast amounts of data and advanced analytics, these algorithms provide manufacturers with valuable insights for optimizing production planning and meeting customer requirements.
Leveraging machine learning algorithms for demand prediction brings numerous advantages to manufacturing operations:
- Enhanced demand forecasting: Using the power of machine learning algorithms, manufacturers can gain a deeper understanding of consumer preferences and anticipate demand more accurately. This enables them to align their production levels, inventory management, and supply chain operations accordingly.
- Streamlined production planning: Exploiting the insights derived from machine learning algorithms allows manufacturers to streamline their production cycles, reduce lead times, and ensure timely delivery of products. This enables them to meet customer demands efficiently while minimizing excess inventory or stockouts.
- Informed decision-making: Analysing consumer behaviour and market trends, machine learning algorithms empower manufacturers to make data-driven decisions regarding product development, marketing strategies, and supply chain management.
In the consumer electronics industry, machine learning algorithms analyse historical data on consumer buying patterns, seasonal trends, and market dynamics to predict demand for specific products. This enables manufacturers to tailor their production output, allocate resources effectively, and stay responsive to consumer needs.
6. AI systems detect errors
Automated visual inspection is a vital component in manufacturing industries, where maintaining high-quality standards is paramount. AI systems, powered by advanced technologies such as machine vision cameras, have revolutionized the defect detection process, enabling manufacturers to enhance their quality control measures and minimize product defects.
Key aspects of AI-based defect detection in manufacturing include:
- Need for automated visual inspection: Traditional manual inspection methods are time-consuming, prone to human error, and limited in their ability to detect subtle defects. AI systems offer a more efficient and accurate alternative, leveraging machine vision cameras and sophisticated algorithms to analyse visual data rapidly.
- Role of machine vision cameras: Machine vision cameras capture detailed images of products, components, or assemblies during the manufacturing process. These images are then processed by AI algorithms, which analyse the visual data to identify defects, anomalies, or deviations from quality standards.
- Improving quality control with AI-based inspection: One notable case study comes from the automotive industry, where AI-powered inspection systems have been deployed to enhance quality control during the production of vehicle components. These systems can identify imperfections, such as surface scratches, dents, or misalignments, with high accuracy and speed, ensuring that only defect-free parts are used in the final assembly.
For instance, a manufacturing company producing electronic devices can employ AI systems to inspect printed circuit boards (PCBs) for any soldering defects, such as misalignments, bridging, or insufficient solder. By automating the inspection process with AI, the company can significantly reduce the risk of faulty PCBs reaching the market, ensuring higher product quality and customer satisfaction.
Future of AI in Manufacturing Industry
As we explore the remarkable advancements in artificial intelligence (AI) within the manufacturing industry, it is important to recognize the rapid pace of change and the potential for this blog to become quickly outdated. The field of AI is constantly evolving, pushing the boundaries of what is possible and driving transformative shifts in the manufacturing landscape.
While we strive to provide valuable insights and a comprehensive understanding of the current state of AI in manufacturing, it is crucial to remain cognizant of the dynamic nature of this technology. The advancements we discuss today may be surpassed tomorrow, and new breakthroughs may reshape the industry in unforeseen ways. Nonetheless, by embracing this reality and staying abreast of the latest developments, we can navigate the future of AI in manufacturing with agility and open ourselves up to the endless possibilities that lie ahead.
Conclusion
The integration of artificial intelligence (AI) in metal manufacturing has transformed the industry, enabling significant advancements in efficiency, productivity, and product quality. We have explored various AI use cases that demonstrate the transformative power of this technology. From collaborative robots (cobots) working alongside human workers to predictive maintenance systems and lights-out factories, AI has reshaped the landscape of component manufacturing.
As the manufacturing industry continues to evolve, embracing AI will be crucial for maintaining a competitive edge. The future holds immense potential for advancements in AI technologies, such as the integration of machine learning algorithms for advanced analytics, the utilization of big data to drive decision-making, and the continued development of robotics and automation. Manufacturers that recognize the significance of AI and proactively adopt these technologies will be well-positioned to achieve operational excellence, deliver high-quality products, and stay ahead in the dynamic market landscape. Embracing AI not only enhances efficiency and productivity but also positions manufacturers for future growth and innovation in the ever-evolving manufacturing landscape.